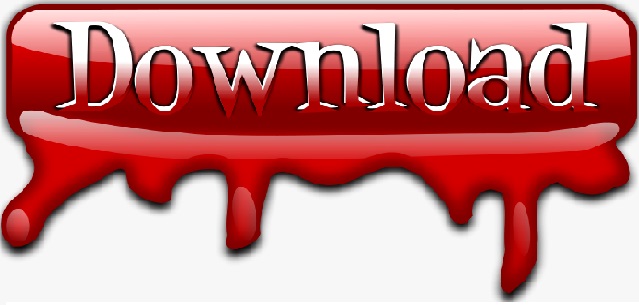
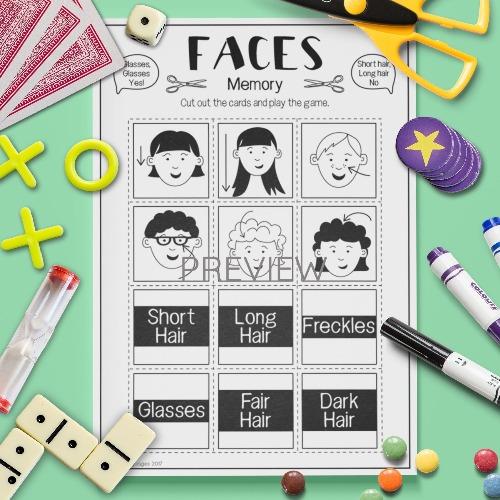
Taking advantage of having full control over the rendering pipeline, we also study how each attribute (e.g., variation in facial pose, accessories, and textures) affects the accuracy.įinally, by fine-tuning the network on a smaller number of real face images that could reasonably be obtained with consent, we achieve accuracy that is comparable to the methods trained on millions of real face images, while alleviating the problems associated with large datasets. We first demonstrate that aggressive data augmentation can significantly help reduce the domain-gap between our synthetic faces and real face images. We compare our method to SynFace, a recent method trained on GAN-generated synthetic faces, and reduce the error rate on LFW by 52.5% (accuracy from 91.93% to 96.17%). To avoid the problems associated with real face datasets, we introduce a large-scale synthetic dataset for face recognition, obtained by photo-realistic rendering of diverse and high-quality digital faces using a computer graphics pipeline. Most importantly, these face images are collected without explicit consent, raising more pressing privacy and ethical concerns. It's at the bottom of the first list of options here. It's in the bottom right corner of the screen. If you aren't signed into Facebook, enter your email address (or phone number) and password and tap Log In.

Web-crawled face images are severely biased (in terms of race, lighting, make-up, etc) and often contain labeling noise. Method 1 Using an iPhone or iPad 1 Open the Facebook app. However, these models are trained on large-scale datasets that contain millions of real human face images collected from the internet. State-of-the-art face recognition models show impressive accuracy, achieving over 99.8% on Labeled Faces in the Wild (LFW) dataset.
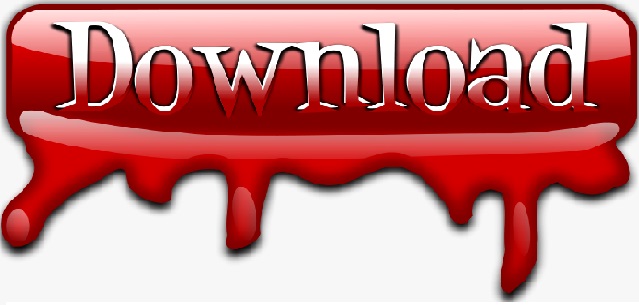